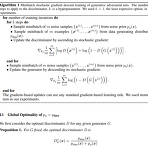
Generative Adversarial Network ■ minimax problem $\underset{G}{min}\, \underset{D}{max} V(D, G) = \mathbb{E}_{x \sim P_{\text{data}}(x)} \big[ \log D(x) \big] + \mathbb{E}_{z \sim P_{z}(z)} \big[ \log (1-D(G(z)) \big]$ ■ Discriminator $maximize\quad J^{(D)}= \mathbb{E}_{x \sim P_{\text{data}}(x)} \big[ \log D(x) \big] + \mathbb{E}_{z \sim P_{z}(z)} \big[ \log (1-D(G(z)) \big]$ $\frac{1}{N}\sum_{..
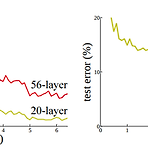
■ Degradation 문제 Deep Residual Learning for Image Recognition 논문을 보면 시작은 56-layer를 사용하면 이론적으로 더 error 가 낮아야 하지만, 20-layer 보다 더 높은 값을 나타내고 있습니다. 이는 다시말하면 layer가 깊어질수록 overfitting 의 문제가 아닌, training error 가 증가한다는 말로 네트워크가 학습이 잘 진행이 되지 않는다는 것을 의미합니다. ■ Shortcut connection (skip connection) Degradation 문제를 해결하기 위해 논문에서 제안한 방법이 shutcut connection 이란 방법으로 Gradient 를 유지할 수 있도록 shorcut을 만든 다는 것이 핵심입니다. ..
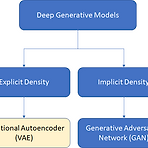
* Variational Autoencoder 생성 모델 중 VAE 정리 진행 중 (영상 대신 1d 시그널 생성 모델) # coding: utf-8 # In[1]: import os import keras import numpy as np import matplotlib.pyplot as plt import tensorflow as tf # In[2]: def sinosoidal(v): fix_wave = np.arange(100) return (fix_wave**0.2)*np.sin(0.3*fix_wave + v) + v # In[3]: spectrum_y = np.random.normal(size=(1000,1),scale= 0.5) spectrum_x = np.vstack([sinosoidal(i[..
- Total
- Today
- Yesterday
- numpy
- DWT-DCT
- SvD
- Residual Block
- 네이버웹툰
- dct
- Digital watermarking
- gPRC
- backpropagation
- tensorflow serving
- flask serving
- keras
- DW
- 캡처방지
- implementation
일 | 월 | 화 | 수 | 목 | 금 | 토 |
---|---|---|---|---|---|---|
1 | 2 | 3 | 4 | |||
5 | 6 | 7 | 8 | 9 | 10 | 11 |
12 | 13 | 14 | 15 | 16 | 17 | 18 |
19 | 20 | 21 | 22 | 23 | 24 | 25 |
26 | 27 | 28 | 29 | 30 | 31 |